Benchmark¶
import os
import numpy as np
import pandas as pd
from tqdm.auto import tqdm
from functools import partial
import matplotlib.pyplot as plt
from commplax import util
from gdbp import gdbp_base as gb, data as gdat, aux
WARNING:absl:No GPU/TPU found, falling back to CPU. (Set TF_CPP_MIN_LOG_LEVEL=0 and rerun for more info.)
aux.dl_trained_params() # download pre-trained parameters
loc_trained_params = './trained_params/pretrained'
100% [..........................................................................] 1582928 / 1582928
LP = np.array([-4, -2, -1, 0, 1]) # launched power in dBm
# use Pandas.Dataframe to store the results
df_test_res = pd.DataFrame({c: pd.Series(dtype=t) for c, t in {'ChInd': 'int',
'LPdBm': 'float',
'Model': 'str',
'Q': 'float'}.items()})
# check `gdbp_study-master/src/gdbp_base.py` for model definition
def init_models(data: gdat.Input, **kwargs):
''' make CDC and DBP's derivatives
(all methods has trainable R-filter)
cdc: static D-filter, no NLC
dbp: static D-filter, scalar manually optimized NLC factor
fdbp: static D-filter, static N-filter scaled by manually optimized NLC factor
edbp: static D-filter, tap-by-tap optimizable/trainable N-filter
gdbp: tap-by-tap optimizable/trainable D-filter and N-filter
'''
mode = kwargs.get('mode', 'train')
steps = kwargs.get('steps', 3)
dtaps = kwargs.get('dtaps', 261)
ntaps = kwargs.get('ntaps', 41)
rtaps = kwargs.get('rtaps', 61)
xi = kwargs.get('xi', 1.1) # optimal xi for FDBP
fdbp_init = partial(gb.fdbp_init, data.a, steps=steps)
model_init = partial(gb.model_init, data)
comm_conf = {'mode': mode, 'steps': steps, 'dtaps': dtaps, 'rtaps': rtaps} # common configurations
# init. func.| define model structure parameters and some initial values | define static modules | identifier
cdc = model_init({**comm_conf, 'ntaps': 1, 'init_fn': fdbp_init(xi=0.0)}, [('fdbp_0',)], name='CDC')
dbp = model_init({**comm_conf, 'ntaps': 1, 'init_fn': fdbp_init(xi=0.15)}, [('fdbp_0',)], name='DBP')
fdbp = model_init({**comm_conf, 'ntaps': ntaps, 'init_fn': fdbp_init(xi=xi)}, [('fdbp_0',)], name='FDBP')
edbp = model_init({**comm_conf, 'ntaps': ntaps, 'init_fn': fdbp_init(xi=xi)}, [('fdbp_0', r'DConv_\d')], name='EDBP')
gdbp = model_init({**comm_conf, 'ntaps': ntaps, 'init_fn': fdbp_init(xi=xi)}, [], name='GDBP')
return cdc, dbp, fdbp, edbp, gdbp
def load_data(ch: int):
''' build pairs of datasets for training and testing '''
ds_train = gdat.load(1, LP, ch, 2)
ds_test = gdat.load(2, LP, ch, 1)
return [(ds_tr, ds_te) for ds_tr, ds_te in zip(ds_train, ds_test)]
def sweep_channel(ch: int,
df_test_res=df_test_res,
use_pretrained_params=False,
save_params=False,
save_subdirname='regular_taps',
model_init_kwargs={}):
''' sweep launched power of given channel '''
util.clear_xla_cache() # release JAX's cache to save RAM
# iterate data of target channel
for i, chds in enumerate(tqdm(load_data(ch),
desc=f'sweeping launch power on Ch#{ch}',
leave=False)):
ds_train, ds_test = chds
models_train = init_models(ds_train, **model_init_kwargs)
models_test = init_models(ds_test, mode='test', **model_init_kwargs)
# iterate models
for j, (model_train, model_test) in enumerate(tqdm(zip(models_train, models_test),
desc='iterating models',
leave=False,
total=len(models_train))):
params_file = os.path.join(loc_trained_params,
'snr_vs_lp',
save_subdirname,
'params_%d_%d_%d' % (ch, i, j)) # params_{ch}_{lp}_{mod}
if use_pretrained_params:
params = util.load_variable(params_file)
else:
# use trained params of the 3rd last batch, as tailing samples are corrupted by CD
params_queue = [None] * 3
# iterate the training steps
for _, p, _ in gb.train(model_train, ds_train, n_iter=2000):
params_queue.append(p)
params = params_queue.pop(0)
if save_params:
util.save_variable(params, params_file)
test_Q = gb.test(model_test, params, ds_test)[0].QSq.total
# collect result
df_test_res = df_test_res.append({'ChInd': ch,
'LPdBm': ds_test.a['lpdbm'],
'Model': model_test.name,
'Q': test_Q},
ignore_index=True)
return df_test_res
# it may take a while to finish
kwargs = {'save_subdirname': 'regular_taps',
'use_pretrained_params': True, # use pretrained parameters to save time
'save_params': False} # save trained parameters after training
for ch in tqdm(1 + np.arange(7), desc='sweeping channels'):
df_test_res = sweep_channel(ch, df_test_res, **kwargs)
df_test_res
ChInd | LPdBm | Model | Q | |
---|---|---|---|---|
0 | 1 | -4.0 | CDC | 7.998095 |
1 | 1 | -4.0 | DBP | 8.031192 |
2 | 1 | -4.0 | FDBP | 8.088200 |
3 | 1 | -4.0 | EDBP | 8.107054 |
4 | 1 | -4.0 | GDBP | 8.152279 |
... | ... | ... | ... | ... |
170 | 7 | 1.0 | CDC | 7.602585 |
171 | 7 | 1.0 | DBP | 7.864725 |
172 | 7 | 1.0 | FDBP | 8.450531 |
173 | 7 | 1.0 | EDBP | 8.609769 |
174 | 7 | 1.0 | GDBP | 8.663154 |
175 rows × 4 columns
# save results
df_test_res.to_csv('benchmark_regular_taps.csv', index=False)
Now we visualize the results by manipulating the results table, see Pandas.Dataframe for instructions.
grp_ch = df_test_res.loc[df_test_res['ChInd'].isin([1, 4])].groupby('ChInd')
for n_ch, g_ch in grp_ch:
fig, ax = plt.subplots(figsize=(5, 3), dpi=300)
for n_mod, g_mod in g_ch.groupby('Model', sort=False):
ax.plot(g_mod.LPdBm, g_mod.Q, '-o', label=n_mod)
ax.legend(loc='upper left')
ax.set_title(f'Ch#{n_ch}')
ax.set_xlabel('Launched Power (dBm)')
ax.set_ylabel('Q-facotr (dB)')
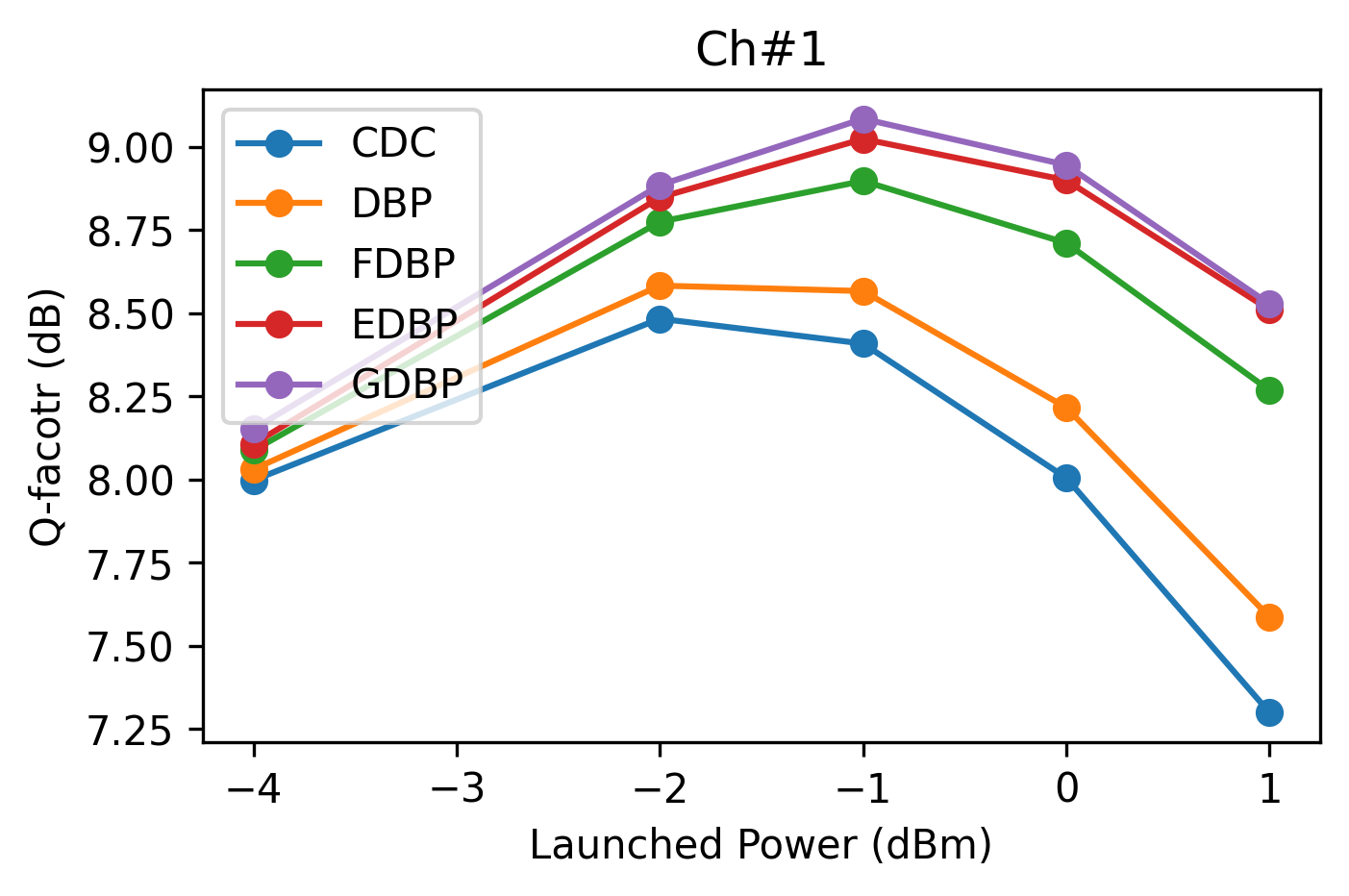
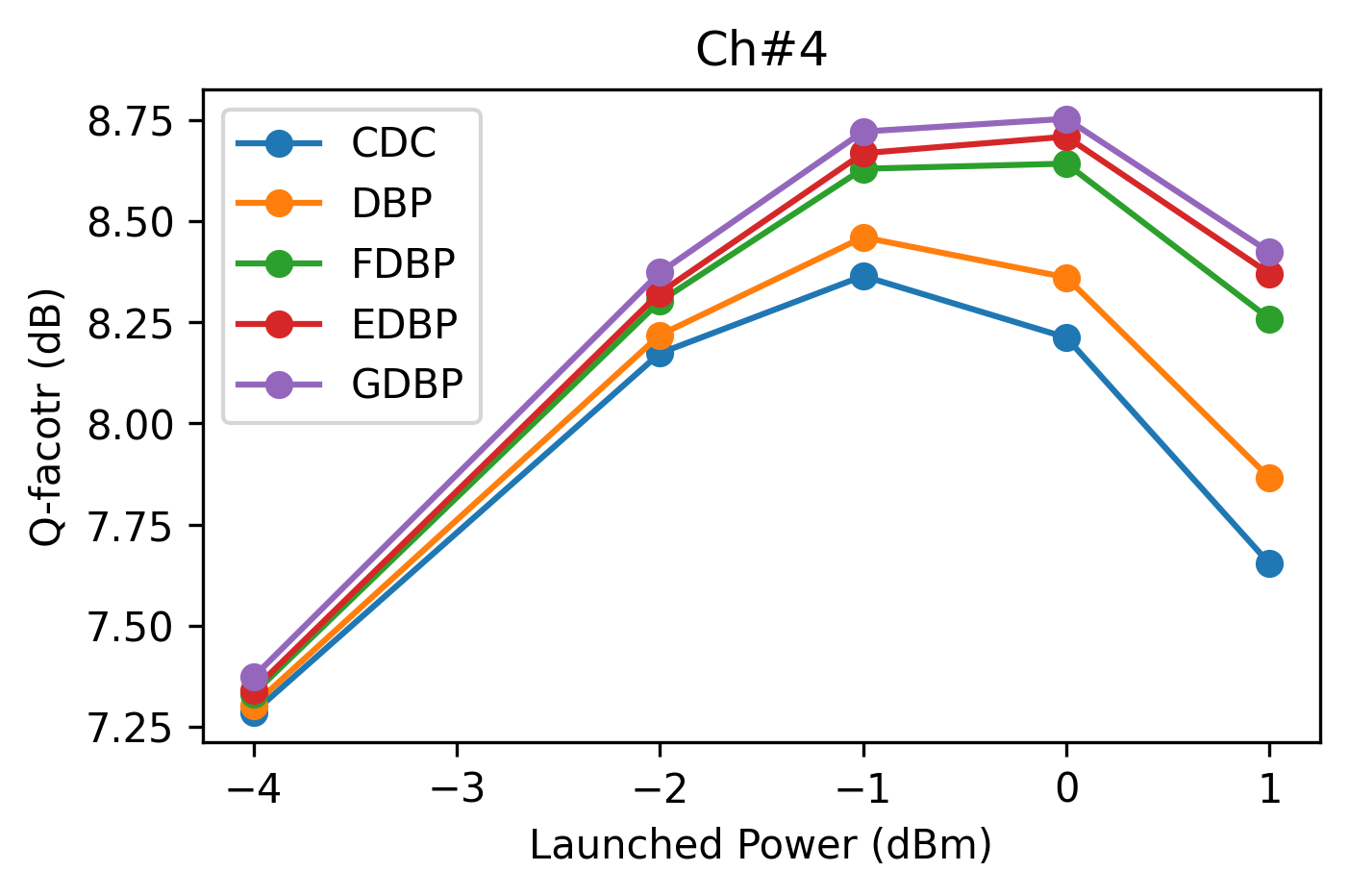
fig, ax = plt.subplots(figsize=(5, 3), dpi=300)
fmt = ['o', '^', '<', 's', '*']
grp_opt_Q = df_test_res.groupby(['ChInd', 'Model'], as_index=False, sort=False)['Q'] \
.max().groupby('Model', sort=False)
for f, (n, g) in zip(fmt, grp_opt_Q):
ax.plot(g.ChInd, g.Q, f, label=n)
ax.legend()
ax.set_xlabel('channel index')
ax.set_ylabel('Q-factor (dB)')
ax.set_ylim([8.15, 9.5])
ax.set_title(r'Optimal $Q$-factor')
Text(0.5, 1.0, 'Optimal $Q$-factor')
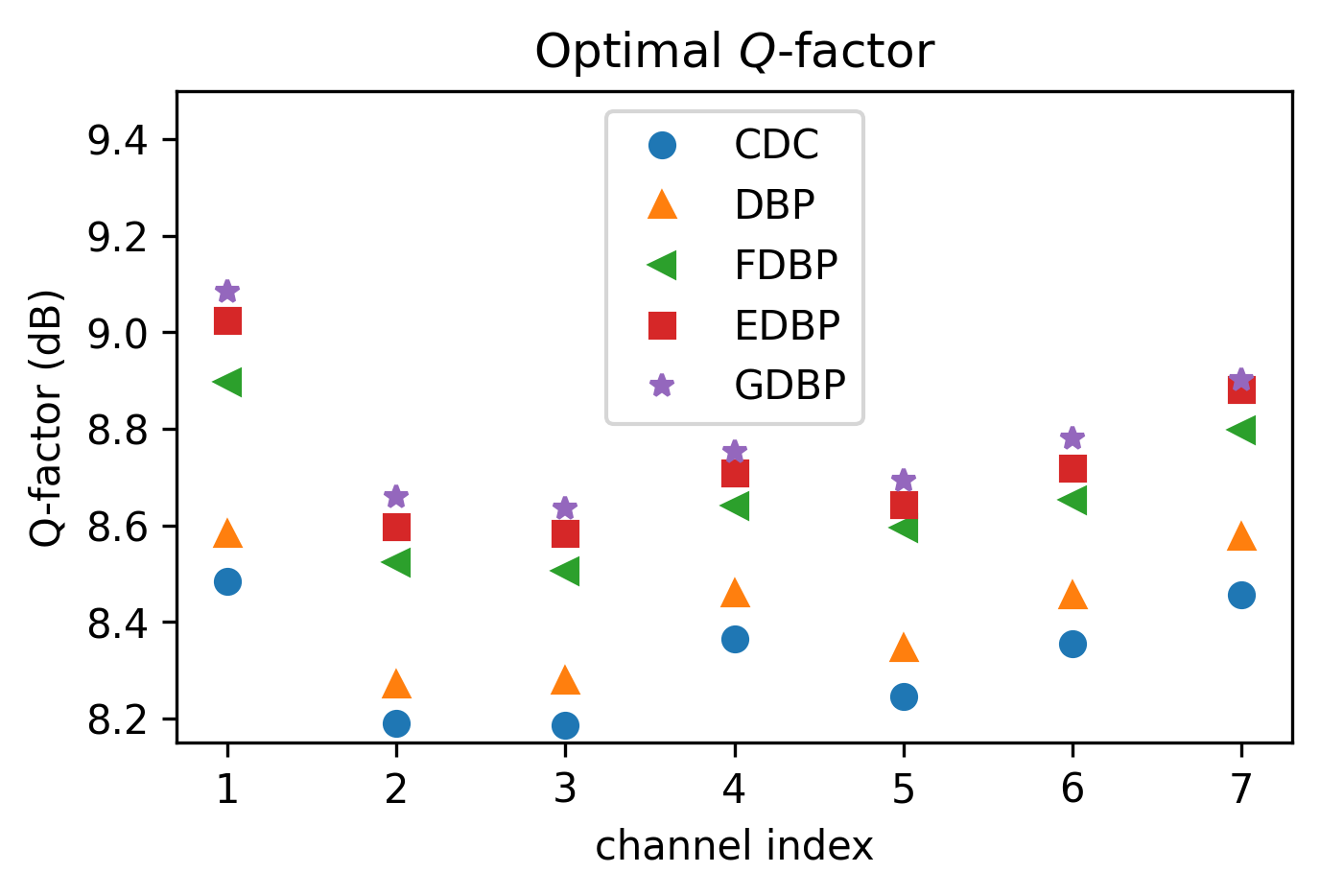
# it may take a while to finish
kwargs = {'save_subdirname': 'few_taps',
'use_pretrained_params': True, # use pretrained parameters to save time
'save_params': False,
'model_init_kwargs': {'dtaps': 221, 'ntaps': 11, 'xi': 0.5}}
df_test_res_ft = sweep_channel(4, df_test_res, **kwargs)
df_test_res_ft
ChInd | LPdBm | Model | Q | |
---|---|---|---|---|
0 | 4 | -4.0 | CDC | 7.102697 |
1 | 4 | -4.0 | DBP | 7.117552 |
2 | 4 | -4.0 | FDBP | 7.124030 |
3 | 4 | -4.0 | EDBP | 7.133463 |
4 | 4 | -4.0 | GDBP | 7.332214 |
5 | 4 | -2.0 | CDC | 7.933425 |
6 | 4 | -2.0 | DBP | 7.982038 |
7 | 4 | -2.0 | FDBP | 8.021433 |
8 | 4 | -2.0 | EDBP | 8.050963 |
9 | 4 | -2.0 | GDBP | 8.322369 |
10 | 4 | -1.0 | CDC | 8.120187 |
11 | 4 | -1.0 | DBP | 8.209987 |
12 | 4 | -1.0 | FDBP | 8.289390 |
13 | 4 | -1.0 | EDBP | 8.337155 |
14 | 4 | -1.0 | GDBP | 8.615173 |
15 | 4 | 0.0 | CDC | 7.973158 |
16 | 4 | 0.0 | DBP | 8.114489 |
17 | 4 | 0.0 | FDBP | 8.236598 |
18 | 4 | 0.0 | EDBP | 8.319276 |
19 | 4 | 0.0 | GDBP | 8.606638 |
20 | 4 | 1.0 | CDC | 7.442021 |
21 | 4 | 1.0 | DBP | 7.645606 |
22 | 4 | 1.0 | FDBP | 7.836688 |
23 | 4 | 1.0 | EDBP | 7.965743 |
24 | 4 | 1.0 | GDBP | 8.212949 |
fig, ax = plt.subplots(figsize=(5, 3), dpi=300)
for n_mod, g_mod in df_test_res_ft.groupby('Model', sort=False):
ax.plot(g_mod.LPdBm, g_mod.Q, '-o', label=n_mod)
ax.legend(loc='upper left')
ax.set_title(f'Ch4 (fewer taps)')
ax.set_xlabel('Launched Power (dBm)')
ax.set_ylabel('Q-facotr (dB)')
Text(0, 0.5, 'Q-facotr (dB)')
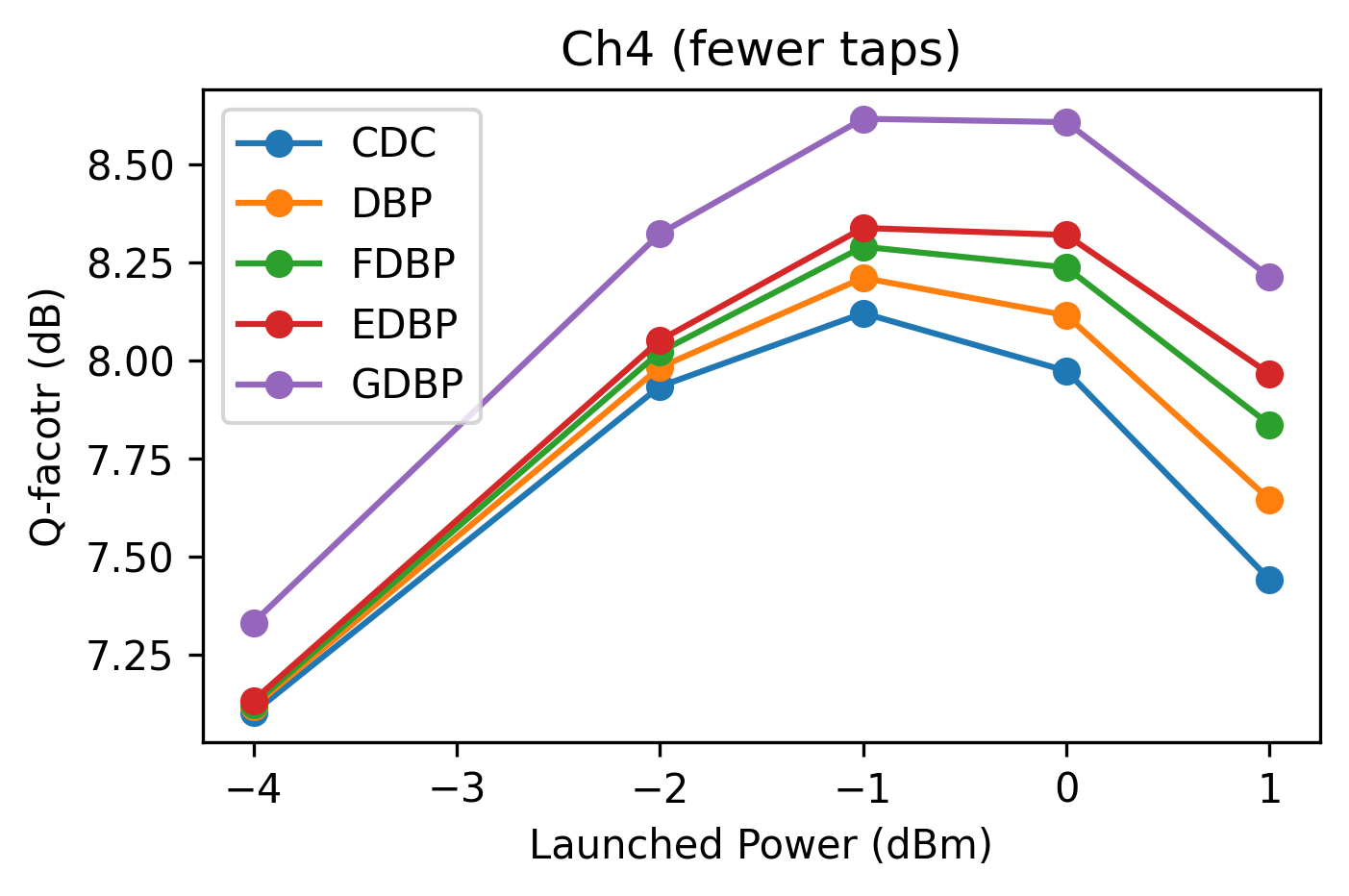